Data Dynasties and Data Governance
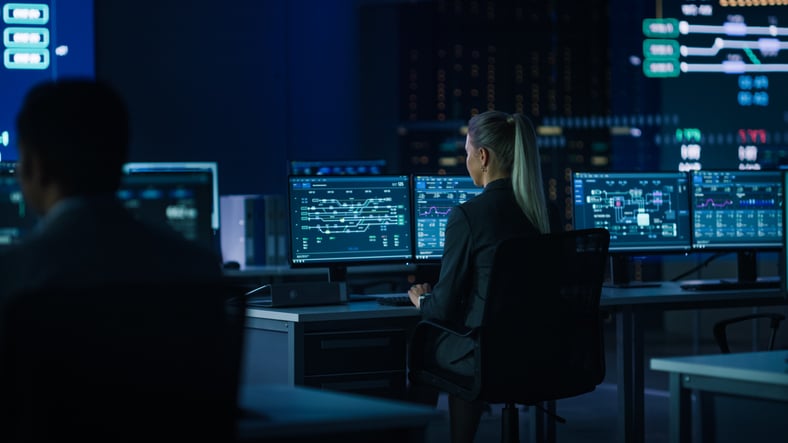
Editor’s note: This is the second article in a series, “Data Disasters Versus Data Dynasties,” authored by data consultants and engineers from IBM’s Procurement Analytics as a Service team. The series will cover common downfalls that procurement organizations make with data, as well as how organizations develop a winning data strategy. The first article can be read here.
***
The role of data governance to an organization is like that of a librarian to your local library. Without a librarian, the library’s books would likely become lost, damaged or difficult to locate.
Likewise, to ensure the safety, quality and accessibility of your enterprise data, you’ll need to depend on a robust data governance program that outlines the policies, procedures and standards that dictate how data is managed throughout the organization.
Consider how a librarian chooses new books to buy for the collection, which sections they should be kept in and who should receive a library card to access those books. Your data governance program answers the same questions about data.
In the procurement world, one-third of companies indicate that access to quality data has a “significant impact” on the speed and accuracy of spend analysis, while one in four companies reports a “very significant impact.” Both the cause of and solution to these data gaps can be traced to an organization’s data governance practices.
The following steps can help you turn your organization’s data governance program into a “data dynasty.”
Governance Best Practices
Despite the wide variety of forms that governance operating models can take (more on this later), successful models are consistently built around a common purpose to coordinate data strategy with business goals. What are the best practices employed by companies with the most mature governance programs?
IBM conducted a study of 1,007 organizations across 18 countries to assess the maturity of their information governance capabilities. Of those that scored among the highest level of maturity, more than half of respondents exhibited the following behavior:
- Implementation of information exchange controls, compliance processes and security awareness programs. Each was reported to be well-known across the organization, regularly reviewed for improvement and measured for user adoption.
- Understanding of the costs of acquiring and maintaining their data. You can create a data roadmap for your organization to identify new datasets to incorporate and their corresponding costs.
- A proven ability to attract, hire and maintain top data and analytics talent. It is noteworthy that the most mature governance programs stand out not just with what governance structures they implement but in how they are implemented. The top-rated governance programs go the extra mile by socializing new initiatives across functions, conducting regular reviews and monitoring the level of adoption. This ensures that these initiatives are being applied in everyday data processes and resulting in a healthier data ecosystem.
A Driver for Advanced Analytics
In partnering with a large global food and beverage company, we found that governance structures were well-established but underperformed in delivering advanced analytics. The most mature data quality organizations are, according to IBM’s study, “2.2 times more likely to have a dedicated program that delivers advanced analytics — including AI (artificial intelligence) and machine learning–organization-wide.”
Through an in-depth assessment of their technology stack and dozens of stakeholder interviews, we found that a major contributing factor to this gap was an inefficient operating model. The various procurement commodity teams were staggered in the areas of use-case development, data access and business intelligence (BI) tool utilization, and they were unaware of reporting functionality and best practices utilized every day by their cross-commodity counterparts.
We recommended an adjusted operating model that prioritized cross-functional knowledge-sharing and procurement expertise. This included a new role be taken on by a procurement analytics leader who has both the functional knowledge to guide strategic use case development as well as an understanding of the data infrastructure and flows required for implementation.
We also identified the need for delegating commodity super users — one to two users from each procurement commodity team who are more technically-minded and can serve as the translator between the procurement analytics leader and the team.
In addition to serving as the first point of contact for technical data questions, the super users could take greater ownership of funneling data requests through the procurement analytics leader, thereby enabling more structured and consistent use-case development for advanced analytics across the enterprise.
Assembling the Team
When conducting an evaluation of your governance operating model, mapping out your optimal structure is a good first alignment step. However, the operating model will only be as effective as the talent and skillsets that support it.
For an efficient procurement analytics team, it’s important to identify these roles:
Technical representation. This is a centralized role or team that will understand the sources of data (in this case ERP data stemming from invoices and purchase orders) and the business intelligence tools used to analyze your data. This could be a centralized enterprise team like roles from the IT, chief information officer or chief data office (CDO) teams or even designated technical representatives within your functional team. Ideally, this team is responsible or knowledgeable about the data governance practices and procedures.
Functional representation. This team would be your usual businesses procurement representatives who are responsible for sourcing, negotiating, or contracting for your business that would benefit from procurement analytics. These could be roles across analysts, buyers, category managers and executives — all of whom would be end users of procurement analytics use cases.
Procurement analytics liaison. This is the most crucial role for a successful procurement analytics program, and also the most difficult role to properly staff. This role acts as the “translator” between the technical and functional teams. The person must have a deep understanding of business problems across the function, from procurement analysts to CPOs.
Procurement super users. These roles are a subset of your functional team, with users by business area (like category-focused, business unit-focused or geo-focused) who are more technically-enabled — and more importantly, technically-curious. These roles will be important in enabling your digital transformation by supporting upskilling your business areas from an analytics perspective. (See graphic.)
Simplified Procurement Data Governance Model
(Courtesy of Benjamin DiPiazza and Brad Ure)
In a simplified operating model, the role of the procurement analytics liaison streamlines communication to the technical representation, thereby allowing for more focused and strategic implementation of strategic analytics.
Please note that the above model is simplified and, although it may not reflect the nuanced structures of an individual organization, it still can be applied. How might this model challenge the way you evaluate the efficacy of your own operating model?
Data Governance That Doesn’t Get in the Way
With an advanced analytics program in full swing, a final consideration is giving end users access to the right data while still maintaining data integrity and security. (As in, who should be granted a library card and to which of the library’s resources?)
In terms of BI tools, the administrators must carefully determine how to govern access to datasets and dashboards. Through role-based access control (RBAC), you can define more granular roles based on job functions to enhance overall security.
For example, an analyst should probably not have access to the dashboards and sensitive information contained within an executive leadership dashboard. This is one way the principle of least privilege may manifest in your data governance strategy.
The principle of least privilege asserts that each user should be limited to the minimum level of data privileges required for their job function. For instance, limiting dashboard users to view-only access on a standard use case is recommended to protect critical data and visuals from being inadvertently modified.
Yet, when the principle of least privilege is taken too far, it can result in excessive regulation of data access. For example, a U.S.-based client in the consumer goods industry had end users unable to copy standard dashboard sets to their workspaces. This inhibited their ability to customize the dashboards to their needs and access critical data fields.
Enabling your end users without sacrificing data governance is a balancing act for your data governance team to manage. The question of which data should be democratized across the enterprise is no exception.
IBM seems to have struck the right balance, with a centralized data management system that dictates which data sets and dashboards are made available for cross-enterprise use. Data made available has been vetted by experts for quality and integrity, enabling users to confidently access it for their projects.
***
An effective data governance team enacts the right policies and procedures to promote the safety, quality and accessibility of its procurement data. A best-in-class data governance team takes it a step further by measuring the impact and adoption of its governance structures, bridging the gap between technical and procurement teams, and empowering users with advanced analytics capabilities. This process accelerates digital transformation and better enables a workforce.
In our next article, we examine how data dynasties are able to maximize the value of their supplier data.