Inside Supply Management Magazine
May/June 2021
Articles
Analytics Analysis: How Data Improves Decision-Making
May 11, 2021
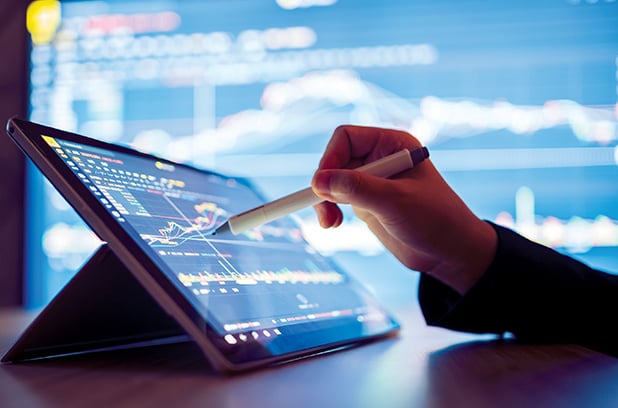
Procurement analytics is designed to provide data-driven solutions to supply management problems. Analytics leverages the organizational capabilities in data management to provide intelligent support for procurement decision-making. Analytics facilitates data collection from several source systems, classifying data to specific taxonomies and visualizing KPIs on dashboards or within business-intelligence tools.
...Want More Magazine?
Oh no... we really want you to have access to all of this great content! Membership does have its benefits and Inside Supply Management magazine is just one piece to help you advance your organization and your career. Learn more and join our community.