Approaching AI With Trust as the Driver
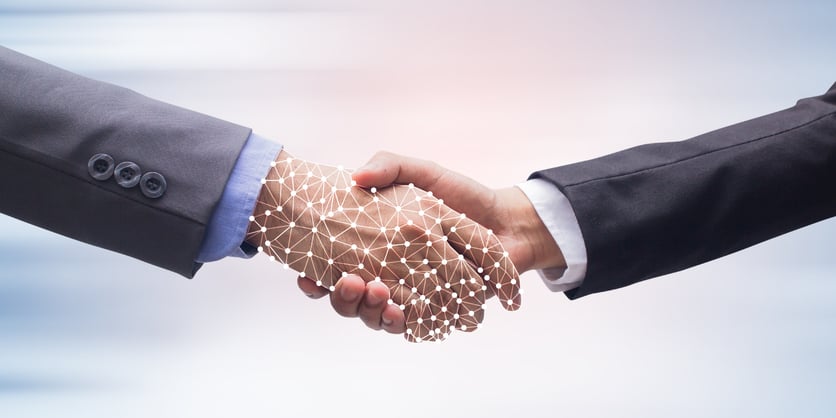
How should a supply management organization approach artificial intelligence (AI) as part of its digital transformation journey? What are the top considerations?
“A lot of the people (are) probably familiar with the feeling that you're building the plane as you're flying it,” said Jeremy Rossi, chief strategy officer and editorial director of Oakland, California-based Leonardo International Society for Arts, Science and Technology. “When you're … bringing in these new tools (like AI), it can feel a little bit like that,” he said, during last week’s “Ensuring Trust in AI: The Importance of Data” webinar hosted by Reuters Events.
Many factors drive a successful AI implementation, panelists said, but behind them all is trust.
Approaching New Technology
For companies enthusiastic about finding a new way to use AI, panelist Jason Urso, vice president and chief technology officer at Honeywell, suggests a framework that considers critical needs, requirements and risks.
First, determine “a critical problem that needs that you're trying to address in the industries you’re serving,” Urso said. In his industry, those problems are, he said: “How do we increase levels of human safety? How do we improve reliability in a large facility where things break? How do we improve human efficiency?”
Once you understand the problem, implement the work process change required to address it, Urso said. For example, it’s important to ensure you have the right data and correct analysis of that data as well as talent with the right skills, so “you can implement a program that allows you to achieve those benefits,” he said.
Having accurate and current data is critical to having the best solution and outcome, said Rouz Tabbador, chief intellectual property (IP) and privacy officer at First American, a Santa Ana, California-based provider of title insurance, settlement services and data analytics.
Inaccurate data is one of the potential risks of using advanced language models. Another pertains to intellectual property. When assessing data, organizations must answer the following questions: Who has access to your IP? Who owns the IP that’s going to be created by AI? How critical is the IP to you?
Laws around AI, including pertaining to copyrights and privacy, are still being formulated, Tabbador said: “The question will be, ‘What are you doing with AI? What kind of IP are you using and what kind of IP are you putting into it and potentially losing rights to?”
Trust is Key
That said, AI use is all about having trust, said panelist Steve McMillan, CEO of Teradata, a San Diego-based multi-cloud data and analytics platform company. “It's got to be a trusted ecosystem that you’re implementing,” he said. “It’s a lot about data and data governance, and the lineage of that data having transparency. It’s also trust from a security perspective.”
McMillan mentioned two other elements critical to any AI implementation. “It’s important to have humans at the center of your AI ecosystem to ensure that there is a moral compass in the AI solutions that are coming through,” he said. Especially in a B2C environment, eliminating bias is critical, he said. Make sure the recommendations that AI offers are accurate, he said, and continuously test them to ensure they’re within your organization’s acceptable parameters.
Additionally, he said, solutions must be sustainable. While much of the current AI conversation is about large language models, “I think you’ll start to see organizations implementing medium-sized language models and small language models that are very focused on certain areas and running in a controlled ecosystem,” he said.
Such language models also offer a level of trust.