Optimizing Demand Forecasting: Challenges and Best Practices
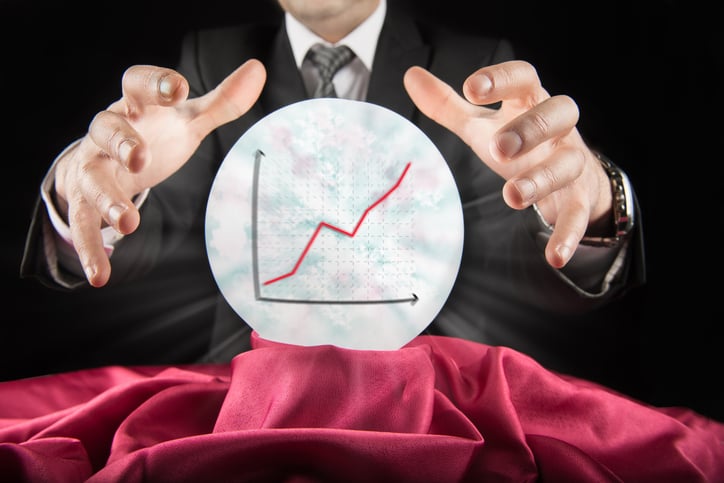
Demand forecasting is a critical aspect of supply management, equipping businesses with the foresight needed to anticipate future product and service demands. The accuracy of these forecasts is key to ensuring optimal inventory levels, efficient resource allocation and heightened customer satisfaction.
However, navigating the complexities of accurate demand forecasting presents challenges. But by integrating industry-specific approaches and forward-looking insights, businesses can improve forecasting accuracy and strategic decision-making in an ever-evolving market landscape.
Challenges in Demand Forecasting
Data inaccuracy and availability are among the biggest challenges for organizations.
Reliable data is crucial for forecasting models, but the lack of accurate historical data can impede the forecasting process. Businesses can address this by implementing data governance practices, conducting regular data audits and using data enrichment techniques.
Incorporating seasonal and cyclical patterns into forecasts is another consideration. Many products and services experience fluctuations in demand throughout the year, and these patterns must be reflected in forecasts. Techniques like seasonal indexing and cyclical analysis can help refine forecasts to account for these variations.
The constant introduction of new products and changes to existing ones can also complicate forecasting. Businesses can use proxy data or pilot studies to predict demand for new products, and gradually phase out forecasts for discontinued products, while closely monitoring them.
In fast-evolving industries, forecasting models can quickly become outdated due to rapid technological advancements. To stay ahead of the curve, businesses need to stay informed about industry trends and technological changes, then update forecasts accordingly.
The product life cycle also presents challenges. New product launches and the phase-out of older products can disrupt established forecasting models. Businesses need to adjust their forecasts to account for these life-cycle stages, by ramping up forecasts for new products and winding down forecasts for older ones.
Common Pitfalls
One common pitfall that can lead to inaccurate forecasting is overreliance on historical data. While such data is valuable, using it alone can lead to inaccurate forecasts, especially in dynamic markets. It’s better to take a more comprehensive approach that combines historical data with current trends and future-oriented indicators.
Other pitfalls include:
- Neglecting external factors like economic conditions, market trends and competitor actions that provide a more holistic view of the market.
- Overly complex forecasting models. While sophisticated models may seem appealing, they can be prone to errors and difficult to interpret. Simpler and clearer models are generally more reliable and easier to use.
- Lack of communication between departments. Businesses should encourage collaboration across departments and ensure all stakeholders are informed and aligned on forecasting strategies.
- Failure to update. Forecasts need to be regularly reviewed and updated to reflect new data and changing market conditions. Businesses should also monitor their forecasts to identify any unexpected changes in demand.
Best Practices
For more effective demand forecasting, businesses can employ a combination of quantitative and qualitative methods, depending on the specific situation.
In addition to regularly reviewing and updating forecasting models, ensure collaboration between the sales, marketing and supply management teams to gain valuable insights. Also, integrate external factors like economic trends, market dynamics and weather patterns into forecasting models. Businesses can further refine their forecasts by leveraging advanced analytics, artificial intelligence (AI) and machine learning.
It is important to remember that no forecasting model is perfect. Businesses need to understand the limitations of their models and incorporate buffers or safety stock to account for potential inaccuracies.
Industry-Specific Approaches
Achieving effective demand forecasting also requires considering industry-specific approaches. In retail, for instance, businesses can leverage point-of-sale (POS) data and customer loyalty program insights and perform basket analysis (of products customers frequently buy together) to predict demand. Additionally, techniques like collaborative planning, forecasting and replenishment (CPFR) involve suppliers in the forecasting process, leading to more accurate predictions.
The health-care industry has its own unique forecasting needs, including predicting patient volume or demand for a medical device or supplies. Advanced algorithms and simulations, integrated with electronic health records (EHR), can play a vital role in developing a better forecast.
For manufacturers that rely on lean manufacturing principles like just-in-time (JIT) inventory, accurate demand forecasts are essential. Integrating ERP systems with forecasting tools can make a difference.
The rise of renewable energy sources has made energy demand forecasting more complex. In this sector, advanced analytics combined with weather pattern data are frequently used to predict demand fluctuations.
Trends to Watch
The field of demand forecasting is constantly evolving, driven by technological advancements and changing market dynamics. Among trends likely to shape the future of demand forecasting:
- Increased integration of artificial intelligence (AI) and machine learning, which are becoming more sophisticated, enabling more accurate and nuanced demand predictions. These technologies can analyze complex patterns in vast data sets, including unstructured data from social media and consumer sentiment analysis.
- Real-time data analysis. The ability to process and analyze data in real-time will become increasingly important. This will allow businesses to react more swiftly to market changes, reducing the time between data collection and decision-making.
- Predictive analytics. Businesses will move beyond traditional forecasting and adopt predictive analytics to anticipate future trends and consumer behaviors. Advanced algorithms will be used to predict not just demand but also potential market disruptions.
- Customization and personalization. As consumer preferences shift towards more personalized experiences, demand forecasting will need to become more granular and individualized.
- A collaborative forecasting approach, in which organizations join with suppliers, customers, and even competitors, will continue to grow, allowing for a more comprehensive understanding of market dynamics.
Strategies to Follow
To navigate these future trends successfully, businesses should consider:
- Embracing technological innovations. Invest in the latest forecasting technologies and tools to stay ahead of the competition.
- Data literacy and workforce training. Cultivate data literacy across the organization and invest in training to use advanced forecasting tools and interpreting their outputs.
- Agility and flexibility. Develop agile and flexible business processes that can quickly adapt to new information and changing market conditions. This includes the capability to scale operations up or down based on real-time demand insights.
- Focusing on collaboration. Foster relationships with all stakeholders in the supply chain. Sharing information and insights can lead to more accurate and comprehensive forecasts.
Demand forecasting is entering a new era characterized by technological sophistication and a greater emphasis on real-time data, predictive analytics and sustainability. By embracing these trends and investing in the necessary tools and skills, businesses can significantly improve the accuracy of their forecasts and their overall responsiveness to market changes.
As demand forecasting continues to evolve, it will play an increasingly vital role in shaping business strategies and operations, driving efficiency and fostering innovation in the dynamic global market. The future of demand forecasting is not only about predicting what will happen, but also about being prepared to adapt quickly and responsibly.