Strategies for Measuring Data Quality
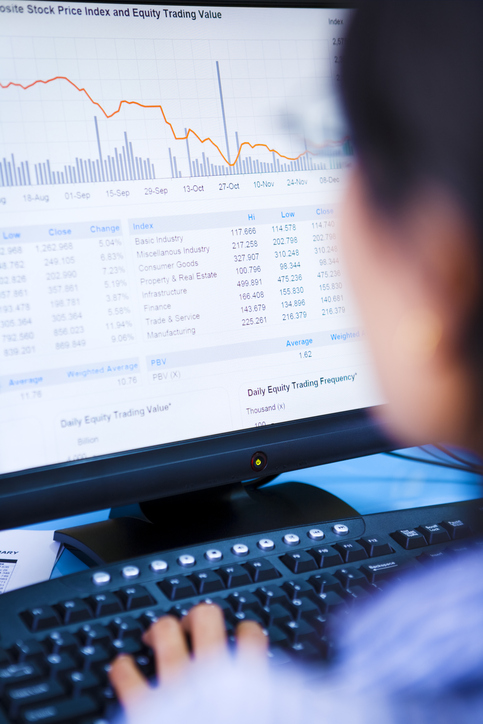
In business and procurement, data is at the center of everything. With each decision or step in a process, a data point is created. Due to the vast amounts of complex and varied data produced daily within organizations, it is important to ensure data is of high quality.
High-quality data is valuable data — organizations with high-quality data can make better informed decisions. Having high-quality data also reduces the risk of errors and creates a competitive advantage as a reputable data-driven organization.
Data Measurement Standards
Data quality is the management of activities and techniques that allow data to best fit the needs of its intended purpose. It refers to the overall ease of utilization of the data for its specific objective as well as additional purposes.
Data quality level can be determined by measuring it, a process dependent upon the situational context and the desired data quality dimensions. Most organizations use specific dimensions to measure and assess data quality.
Six core dimensions are widely recognized as the standards for data quality measurement:
- Accuracy: How does the data compare to reality or the verified source?
- Completeness: Does the data deliver a comprehensive view of the available values?
- Consistency: Does the data offer uniformity when coming from different sources?
- Timeliness: Is the data available when it is required?
- Validity: Does the data adhere to the business defined rules while conforming to the proper format?
- Uniqueness: Does the data have duplicates?
These dimensions can be adjusted to fit the organization’s goals. To do so, an organization generally will weight dimensions by prioritizing them to achieve an accurate and organization-specific data quality measure. Then, a data quality assessment can be conducted; it can serve as a baseline for the measurement of data quality and as the foundation for implementing improvement initiatives.
It's important to have a starting point to understand the current level of quality and utilize this to determine the target goal.
Additional Strategies
Once a measurement system has been defined and a baseline has been established, an organization can utilize several different strategies to improve its data quality, including:
Implement data profiling, which is the process of utilizing algorithms, statistical tools or business rules to analyze source data to better understand underlying relationships and structures. It is an essential and iterative process that helps aid in the data-quality issue exploration and provides an overall summary of data. It can be useful when looking to identify inaccuracies, inconsistences or duplications within the data.
Overall, data profiling is a great way to kick start a data-quality improvement initiative because it provides valuable insights that drive key organizational advancements.
Perform data quality monitoring, the process of routinely checking data for each instance that is created against a defined set of business rules or parameters. This will ensure that a high standard of quality is met.
Data quality monitoring can be performed through the use of preset algorithms or machine learning; most have the ability to detect and send an alert if the quality rules and parameters are violated. For many organizations, data quality monitoring saves on time and cost because it allows the data to be checked while it is being created rather than while it is being analyzed or processed.
Promote a data-driven culture. The first step to is to make data easily accessible. Establish a good warehouse to store data and grant access to employees. A data-driven culture starts with those in charge: Executives and leaders should initiate data-driven decisions and processes; in doing so, their practices will be reflected to the rest of the organization.
A key to a data-driven culture is strengthening the data skill set of those using data. Classes, including free online courses, can help professionals learn the skills needed for working with data.
Benefiting from High-Quality Data
Organizations that have high data quality can make more informed decisions — leading to higher growth and improved efficiency and productivity within the organization.
Poor data quality usage, on the other hand, comes with such risks as low model accuracy, low customer trust and poor decision making. As data quality improves, these risks can be reduced.
Data can be thought of as the ingredients of a recipe. With outdated or incorrect ingredients, the recipe will never be as good as it is meant to be. Essentially, when there is high data quality, every entity that uses that data will be enabled with the correct ingredients to make a valuable recipe.
A quick tip: Improving data quality is not a one-time activity, but a continuous and iterative process that takes time to realize organization-wide results. Keep in mind the various areas within an organization that data can seep into and understand that while there will be obstacles along the way, the process is well worth the results.